By Chet Babla, SVP Strategic Marketing
With driver assistance and driving automation requiring multiple sensors and real-time processing, ADAS electrical and electronic system architectures will be challenged by scalability.
The societal benefits of greater driver, passenger and road user safety are well understood and documented, and indeed governments are increasingly regulating the need for advanced driver assistance systems (ADAS) in new vehicles (see example). In addition to the obvious human impact of safety systems, a recent McKinsey report estimates that ADAS already represents a $55-70 billion revenue opportunity today, and in combination with autonomous driving (AD) could collectively represent a $300-$400 billion revenue by 2035 (see figure 1).
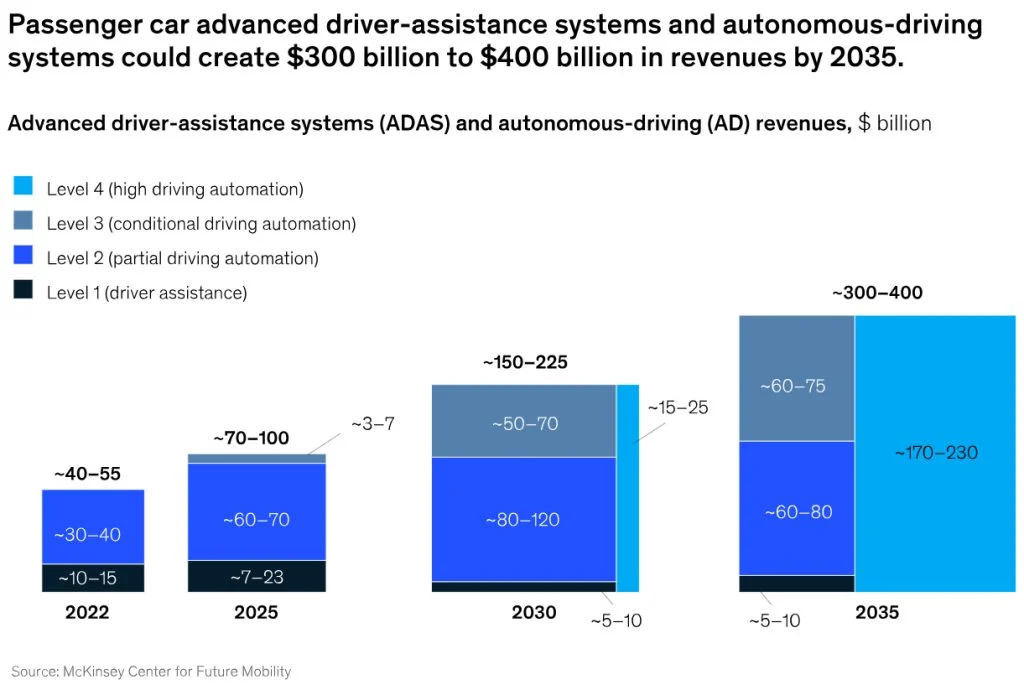
Figure 1 – ADAS and AD revenues in $ billions (source McKinsey Center for Future Mobility)
To support the functionality and safety goals needed for higher levels of automation, the number of sensors on the vehicle and the ability to process data in real-time, will need to increase greatly (refer to our blog In Pursuit of the Uncrashable Car).
Both ADAS and AD leverage various sensor modalities (including computer vision, ultrasonic, radar and LiDAR; see also our Blog discussing these) to gather and process data about the vehicle’s exterior and interior environment.
In terms of ADAS sensing, multiple trade-offs exist. For example, radar provides good sensing ability of objects crossing or coming into a vehicle’s path across a range of weather conditions but has limited depth precision and object recognition capability. Conversely, cameras are extremely capable for object recognition in the same way vision sensing is for humans but have poor performance in adverse weather or lighting conditions. And while LiDAR excels in range and depth precision, and is unaffected in poor lighting, it may be impeded by heavy rain or fog.
Dissecting the Sensing System Architecture
Moreover, the need for sensor fusion – combining the information from different sensor modalities – is well recognized as critical for enhanced perception and decision-making capability.
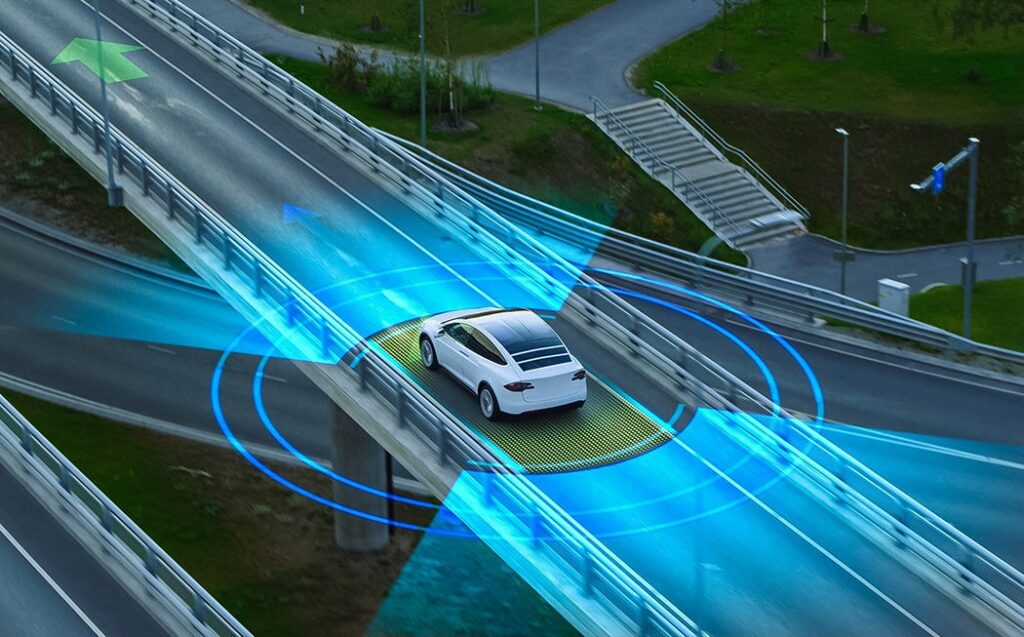
The sensing system architecture is therefore a critical factor in the overall achievable ADAS and automation level capability and determining the practical constraints that will be imposed upon the system designer in terms of power, size, and cost. A recently published article (‘How Many Sensors For Autonomous Driving?’) reviews some of these sensor considerations and challenges, and observes:
“When finally implemented, Level 3 autonomous driving may require 30+ sensors, or a dozen cameras, depending upon an OEM’s architecture.”
This sensor proliferation is a challenge not just affecting Level 3 automation. Indeed, several premium vehicles already feature upwards of 30 sensors across multiple modalities for Level 2 and 2+ ADAS capability. Consider the case of camera sensing. Robust and real-time object detection of lane markings, traffic signs, pedestrians etc., requires multiple cameras with up to 12-megapixel resolution. These sensors will generate large quantities of imaging data that needs to be transported and processed and, depending upon the ADAS electrical and electronic (E/E) processing architecture, may lead to data bottlenecks and other significant system design challenges.
Another recent McKinsey article explores through a series of hypotheses the growing trends in automotive E/E architectures – including factors driving hardware consolidation into fewer electronic control units (ECU) leveraging monolithic silicon integration – and captures many of the key industry drivers for architectural change. In addition to the McKinsey article’s observations, there has been a growing narrative over the last few years that a “Central Compute” ADAS architecture will ultimately become the de facto industry approach. This narrative has especially been supported by some entrant ‘clean sheet architecture’ EV-only players, Robotaxi companies and premium class-only OEMs – all having either no legacy platforms or only a very small number of platforms to support. However, indie believes that while vehicle ECU consolidation is absolutely the right direction for the industry, the Central Compute ADAS architecture assertion cannot be applied as a one-size-fits-all as it overlooks the needs of the majority of OEMs for vehicle platform scalability. Scalability is better achieved through a “Distributed Intelligence” ADAS architectural approach.

In a Central Compute sensing architecture, raw multi-sensor data is typically transported directly to a centralized system-on-chip (SoC) processor, and little to no processing at the edge is employed. The central compute SoC ingests and processes the raw sensor data to perform environmental perception, leveraging powerful AI algorithms to control the vehicle’s motion-related actuations. Such a central compute architecture can confer theoretical benefits related to vehicle software management and updateability and leverages early fusion-based perception for potentially richer algorithmic processing. However, it also necessitates very high sensor-to-processor interface bandwidth and significant computing processing power and memory. In turn, these requirements lead to higher cabling costs and weight, and also present significant thermal management challenges to system integrators dealing with dissipating many hundreds of watts of consumed power in the central compute SoC and supporting infrastructure. Lack of scalability is therefore the Achilles heel for Central Compute.
In a Distributed Intelligence sensing architecture, varying degrees of sensor data processing can be performed closer to the sensors at the vehicle edge deploying SoCs that require relatively modest processing power. The processing performed at the edge can span from full object classification and detection (implying a late fusion perception architecture) to simpler data pre-processing and reduction to ease transport challenges. In the case of camera-based vision sensing as an example, latest use cases (e.g., rear view, surround view) require both human viewing and computer vision-based perception capabilities to address evolving safety requirements (such as Euro NCAP’s vulnerable road user protection protocols).
Such real-time perception can readily be performed at the sensor level, and the resulting pre-processed data aggregated into zones for further downstream processing and perception. This pre-processing reduces the transported sensor data volume, helping to alleviate some of interfacing, cabling and thermal challenges noted previously. This approach – which leverages late(r) fusion – allows automakers the flexibility of city-to-luxury-car platform scalability, sensor optionality and interoperability. Of course, there are trade-offs with a distributed approach related to software management and power distribution towards the edge, and so each OEM must find the right balance of how much pre-processing is performed at the edge, zone or center, aligned to their wider platform needs.
This central versus distributed sensing architecture trade-off is something indie recognized several years ago and we continue to invest and expand our approach to platform scalability, developing edge-based vision sensing and perception solutions that enable distributed intelligence.
The benefits of a distributed sensing architecture are being revisited by the automotive industry which is realizing that a central compute-based sensing architecture may not be a panacea for all. As a result, there are multiple silicon vendors, Tier 1s and OEMs now investigating how selective pre-processing of sensor data within the context of Distributed Intelligence across zones, domains and centralized processing can ease system design, integration and cost challenges.
indie is committed to supporting the industry with an innovative ADAS solutions roadmap, empowering system integrators and OEMs to make the appropriate architectural choices for their platform needs.